Amino acid residues highlighted by the DeepECtransformer neural network. Credit: Natural communications (2023). DOI: 10.1038/s41467-023-43216-z
While E. coli is one of the most studied organisms, the function of 30% of the proteins that make up E. coli has not yet been clearly revealed. For this, artificial intelligence was used to discover 464 types of enzymes from unknown proteins, and the researchers then verified the predictions of three types of proteins successfully identified through an in vitro enzyme assay.
A joint research team, including Gi Bae Kim, Ji Yeon Kim, Dr. Jong An Lee, and Distinguished Professor Sang Yup Lee from the Department of Chemical and Biomolecular Engineering at KAIST, as well as Dr. Charles J. Norsigian and Professor Bernhard O Palsson of the UCSD Department of Bioengineering developed DeepECtransformer, an artificial intelligence capable of predicting enzyme functions from protein sequence. Additionally, the team established a prediction system using AI to quickly and accurately identify the function of the enzyme.
The team’s work is described in the paper titled “Functional annotation of enzyme-encoding genes using deep learning with transformer layers.” The document was published on November 14 in Natural communications.
Enzymes are proteins that catalyze biological reactions, and identifying the function of each enzyme is essential to understanding the various chemical reactions that exist in living organisms and the metabolic characteristics of those organisms.
The Enzyme Commission (EC) number is a classification system of enzyme functions designed by the International Union of Biochemistry and Molecular Biology. In order to understand the metabolic characteristics of various organisms, it is necessary to develop technology that can rapidly analyze enzymes and EC numbers. enzymes present in the genome.
Various deep learning-based methodologies have been developed to analyze features of biological sequences, including protein function prediction, but most of them encounter the black box problem, in which the process of The AI inference cannot be interpreted.
Various prediction systems using AI to predict enzyme function have also been reported, but they do not solve this black box problem or cannot interpret the reasoning process at a finer level (e.g., residue level of amino acids in the enzyme sequence). ).
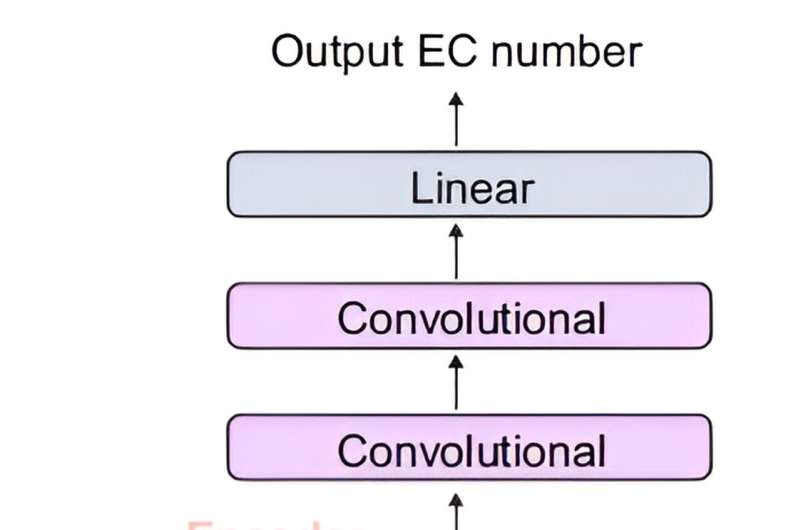
The structure of the DeepECtransformer artificial neural network. Credit: Korea Advanced Institute of Science and Technology (KAIST)
The joint team developed DeepECtransformer, an AI that uses deep learning and a protein homology analysis module to predict the enzyme function of a given protein sequence.
To better understand the features of protein sequences, the transformer architecture, commonly used in natural language processing, was also used to extract important features about enzyme functions in the context of the entire protein sequence, allowing the team to accurately predict the EC. enzyme number. The developed DeepECtransformer can predict a total of 5,360 EC numbers.
The joint team then analyzed the transformer architecture to understand the inference process of DeepECtransformer and discovered that in the inference process, the AI uses information about catalytic active sites and/or binding sites cofactors that are important for the functioning of the enzyme. By analyzing DeepECtransformer’s black box, it was confirmed that the AI was able to identify features important for the enzyme’s functioning on its own during the learning process.
“Using the prediction system we developed, we were able to predict functions of enzymes that had not yet been identified and verify them experimentally,” said Gi Bae Kim, the first author of the paper.
“By using DeepECtransformer to identify previously unknown enzymes in living organisms, we will be able to more precisely analyze various facets involved in the metabolic processes of organisms, such as the enzymes required for the biosynthesis of various useful compounds or the enzymes necessary for the biodegradation of plastics,” he added.
“DeepECtransformer, which quickly and accurately predicts enzyme functions, is a key technology in functional genomics, allowing us to analyze the function of entire enzymes at the systems level,” said Professor Sang Yup Lee.
He added: “We will be able to use it to develop environmentally friendly microbial factories based on comprehensive genome-scale metabolic models, potentially minimizing missing information on metabolism. »
More information:
Gi Bae Kim et al, Functional annotation of enzyme-encoding genes using deep learning with transformer layers, Natural communications (2023). DOI: 10.1038/s41467-023-43216-z
Provided by Korea Advanced Institute of Science and Technology (KAIST)
Quote: Researchers build enzyme discovery AI (November 24, 2023) retrieved November 24, 2023 from
This document is subject to copyright. Apart from fair use for private study or research purposes, no part may be reproduced without written permission. The content is provided for information only.