Perception pipeline: (a) acquisition of RGB and depth images; (b) person detection via Yolact++ (see Sect. III-A); (c) person re-identification using deep neural network and distance between human features; (d) localization of the person using the point cloud and the re-identified person mask; (e) gesture detection to send commands to the robot. Credit: Rollo et al.
In recent years, roboticists and computer scientists have introduced various new computational tools that have the potential to improve interactions between robots and humans in real-world settings. The overarching goal of these tools is to make bots more responsive and responsive to the users they support, which could in turn facilitate their widespread adoption.
Researchers from Leonardo Labs and the Italian Institute of Technology (IIT) in Italy recently introduced a new computational framework that allows robots to recognize specific users and follow them in a given environment. This framework, presented in an article published as part of the 2023 IEEE International Conference on Advanced Robotics and its Social Impacts (ARSO)allows robots to re-identify users in their environment, while performing specific actions in response to hand gestures made by users.
“We aimed to create a groundbreaking demonstration to attract stakeholders to our labs,” Federico Rollo, one of the researchers who carried out the study, told Tech Xplore. “The person tracking robot is a widespread application in many commercial mobile robots, especially in industrial environments or to assist individuals. Typically, these algorithms use external Bluetooth or Wi-Fi transmitters, which can interfere with other sensors and the user is forced to do so. to carry.”
The main goal of Rollo and colleagues’ recent work was to create a re-identification model capable of recognizing specific targets in images recorded by an RGB camera. RGB cameras are among the most used sensors in the field of robotics, so they are very easy to find and integrate into existing robotic systems.
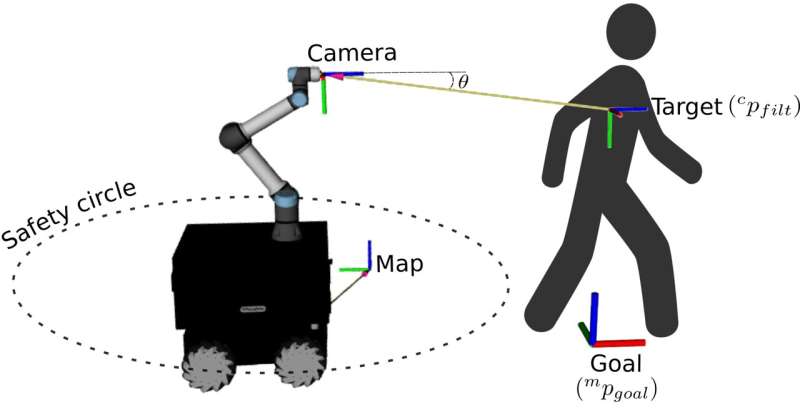
Overview of relevant transformation frames and representation of the safety circle used when applying FollowMe. Credit: Rollo et al.
“The re-identification module we developed includes two consecutive stages: a calibration stage and a re-identification stage,” explained Rollo.
“During the calibration stage, the target person is asked to move randomly in front of the robot. In this phase, the robot uses a neural network to detect the person and know their appearance in the form of embeddings (think of an abstract vector representing the person’s characteristics). These embeddings are then used to create a statistical model that represents the target.
In the second stage of its processing, the module created by the researchers re-identifies the targets as they move naturally through their environment. The framework achieves this by analyzing images acquired by one or more RGB cameras, detecting people in these images, calculating their characteristics and comparing these characteristics with those described in a model of the target user created during the d ‘calibration.
“If certain characteristics statistically match the model, the person with those characteristics is selected as the target,” Rollo said. “This information is then sent to a localization module, which calculates the 3D position of the target user and sends speed commands to the robot to move towards them. Additionally, the application includes a detection module gestures.”
The gesture detection model created by Rollo and colleagues detects specific hand gestures of a target user and sends commands to the robot aligned with those gestures. For example, if a user places an open hand in front of the robot’s field of vision, this triggers the stop command, telling the robot to stop. Conversely, if the user presents a closed hand, the robot will start working again.
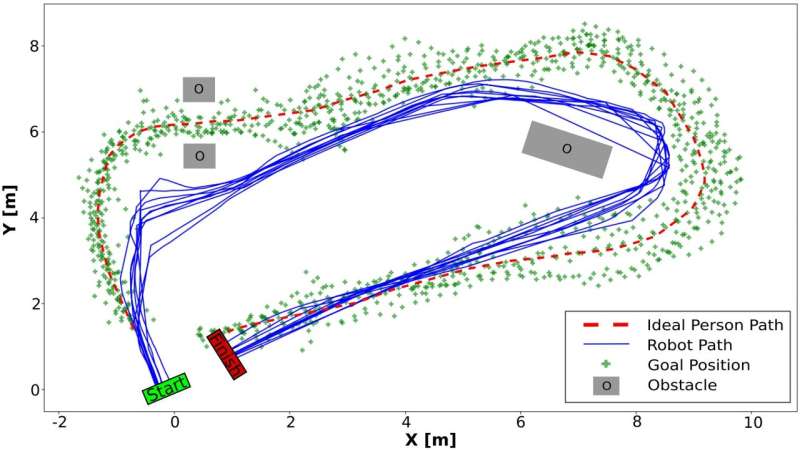
Results of the FollowMe experiment: each person must follow an ideal path (red dotted line) while the robot (blue line) must follow it. Goal positions calculated from perception module data are represented by green plus signs. The robot is placed in the green starting position and must follow the target until the red finishing position is reached. Credit: Rollo et al.
So far, the researchers have tested their framework in a series of experiments using the Robotnik RB-Kairos+ robot. It is a mobile robotic manipulator designed to be primarily introduced into industrial environments, such as warehouses and manufacturing sites.
“The re-identification module demonstrated remarkable robustness during testing, even in busy areas,” Rollo said. “This robust behavior opens up various practical applications. For example, it could be used to move heavily loaded objects in an industrial environment, guide a robot to different stations in a collaborative or industrial environment, or help elderly people move their belongings to the industrial environment. home.”
The new gesture re-identification and detection framework developed by this team of researchers could soon be applied and further tested in various real-world scenarios that require mobile robots to follow humans and transport objects autonomously. Before it can be deployed on a large scale, however, Rollo and his colleagues plan to overcome certain limitations of the model identified during their first experiments.
“A notable limitation is that the statistical model acquired during the calibration phase remains constant during re-identification,” Rollo added.
“This means that if the target changes appearance, for example by wearing different clothes, the algorithm is unable to adapt and requires recalibration. Additionally, there is expressed interest in exploring new approaches to adapt the neural network itself in order to recognize the target, potentially taking advantage of continuous learning methods. This could improve the statistical correspondence between the target model and the features extracted from RGB images, thus providing a more adaptive and flexible.
More information:
Federico Rollo et al, FollowMe: a robust person tracking framework based on visual re-identification and gestures, 2023 IEEE International Conference on Advanced Robotics and its Social Impacts (ARSO) (2023). DOI: 10.1109/ARSO56563.2023.10187536. On arXiv: DOI: 10.48550/arxiv.2311.12992
arXiv
© 2023 Science X Network
Quote: A new model that allows robots to re-identify and track human users (December 11, 2023) retrieved on December 12, 2023 from
This document is subject to copyright. Apart from fair use for private study or research purposes, no part may be reproduced without written permission. The content is provided for information only.