Artist’s impression of a black hole. This representation includes a disk of superheated material that is pulled by the gravitational field, as well as jets of material projected perpendicular to the disk. These jets shine brightly in radio frequencies, a signal that the authors of this study are able to predict from automatic analysis of astronomical images using machine learning techniques. Source: eso.org/public/portugal/images/eso2305b/. Credit: S. Dagnello (NRAO/AUI/NSF)
As far as the eye can see, galaxies fill the images of the deep universe. What processes determined their shapes, colors and star populations? Astronomers believe that primordial black holes were the driving forces behind the growth and transformation of galaxies and may explain the cosmic landscape we see today.
Future sky surveys with radio telescopes will capture millions of galaxies in the early universe, but only automatic tools, like the algorithm created by a team led by the Institute of Astrophysics and Space Sciences (IA) , will be able to read this deluge of data and find the galaxies. with huge black holes at their hearts.
In an article published today (December 6) in the journal Astronomy and astrophysicsan international team led by Rodrigo Carvajal, from the Institute of Astrophysics and Space Sciences (IA) and the Faculty of Sciences of the University of Lisbon (Ciências ULisboa), presents a machine learning technique that recognizes galaxies superluminaries of the first universe.
These are galaxies thought to be dominated by the activity of a voracious black hole at their heart. According to the authors, this should be the first algorithm that predicts when this activity also emits an intense signal in radio frequencies. Radio broadcasts are often distinct from other lights in the galaxy, and it is sometimes difficult to connect them. This artificial intelligence technique will allow astronomers to be more efficient in the search for so-called radio galaxies.
The algorithm, developed in collaboration with the company Closer, operating in the sector of technological solutions for data science, was trained with images of galaxies obtained in several wavelengths of the electromagnetic spectrum. When tested with other images, it was able to predict four times more radio galaxies than conventional methods using explicit instructions.
As machine learning develops its own algorithms, trying to understand its success could help clarify the physical phenomena that were occurring in these galaxies 1.5 billion years after the Big Bang, i.e. when the universe was a tenth of its current age.
“We need to find more active galaxies in the sky, because some predict that there should be many more in the early history of the universe. With current observations, we don’t have that number,” says Carvajal . According to this researcher, more observations are needed to verify whether the current understanding of the evolution of active galaxies is correct or needs to be modified.
“It is also important to analyze the machine learning models themselves and understand what is happening inside,” adds Carvajal. “Which features are most relevant to the decision? For example, we want to know if the most important feature for which the module declared it to be an active galaxy is the light that the galaxy emits in the infrared, perhaps an indication of rapid movement.” formation of new stars. Thanks to this, we are able to produce a new law to separate what is a normal galaxy and an active galaxy.
The relative weight of galactic characteristics on the decision taken by the computer can indicate what is at the origin of its intense activity, particularly in the radio band. In a study in preparation, Carvajal explores the implications of this apparent dependence between radio emission and star formation.
Israel Matute, from IA et Ciências ULisboa, the second author of the article, explains: “These models are mathematical tools that help us look in the right direction when the complexity of the data increases. This work could provide insight into the processes that held back the formation of new stars in the second half of the universe’s history. »
Galaxies that appear to be missing from the early universe could feature in the large body of data that modern radio telescopes will produce in the years to come. Future studies of large regions of the sky will reveal billions of galaxies. One example is the Evolutionary Map of the Universe (EMU), which will map the entire southern celestial hemisphere with the ASKAP radio telescope in Australia.
The AI-led team is already working with data from a pilot of this survey. Once perfected, these tools will be crucial for processing the astronomical amount of data that the future Square Kilometer Array Observatory (SKAO) will produce. Portugal is a member of the consortium of this observatory, already under construction.
“In a new era where astronomy will have access to large amounts of data, it is increasingly important to develop advanced techniques for their processing and analysis,” says José Afonso, of IA and Ciências ULisboa and co-author of this article. “At IA, we develop and implement these techniques, to be able to decipher the origin of galaxies and the supermassive black holes that most of them host.”
The idea of the collaboration between the company Closer and AI was put forward by one of the co-authors, Helena Cruz, who holds a Ph.D. in physics and is a data scientist at Closer. His involvement was essential in analyzing and addressing the impact of uncertainties and inconsistencies between different data sources, from multiple telescopes and observing programs, used to train the machine learning algorithm.
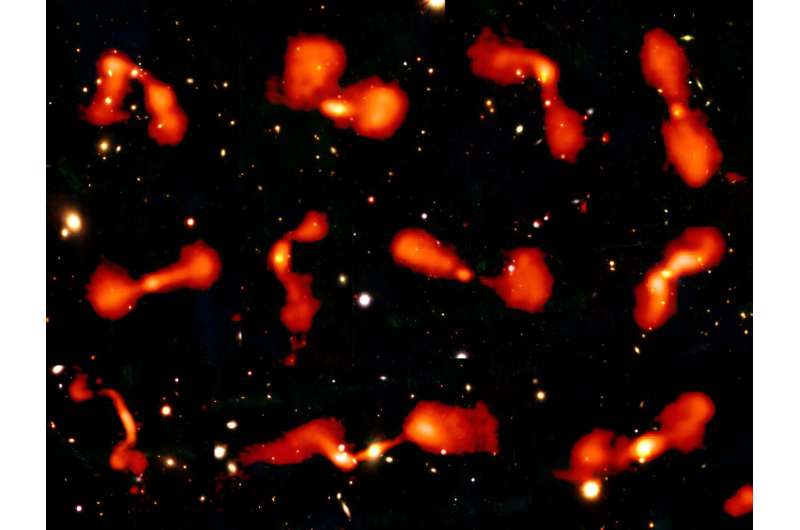
Set of radio galaxies — galaxies with significant radio frequency emission — observed with the LOFAR radio telescope, superimposed on the same region of the sky in visible light. The extent of the radio emission is obvious, distinct from the visible part of the galaxy. These are some of the galaxies used in training the machine learning algorithm developed by this research team. Credit: Judith Croston and the LOFAR investigation team
“I realized that astronomy is a field with great opportunities for the exploration and development of machine learning models, and it seemed logical to me to apply my professional skills to this field,” explains Cruz. “I shared my interest with Closer and both parties immediately showed a willingness to collaborate, which I see as an extension of my work at the company.”
“Closer thrives thanks to the knowledge of its collaborators, it is its capital,” adds João Pires da Cruz, co-founder, professor and researcher of Closer. “The more ambitious and scientifically sophisticated the projects our team members are involved in, the greater the company’s capital. We will have collaborators capable of solving our customers’ problems that are similar to the problem of signals from distant galaxies.
More information:
R. Carvajal et al, Selection of powerful radio galaxies with machine learning, Astronomy and astrophysics (2023). DOI: 10.1051/0004-6361/202245770
Provided by the Institute of Astrophysics and Space Sciences
Quote: Black holes are missing in the early universe and computers are chasing them (December 6, 2023) retrieved on December 7, 2023 from
This document is subject to copyright. Apart from fair use for private study or research purposes, no part may be reproduced without written permission. The content is provided for information only.